
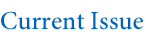
Volume 32, Number 4 (2024)
System Metamodeling of Open-Ended Evolution Implemented with Self-Modifying Code
Patrik Christen
Having a model and being able to implement open-ended evolutionary systems is important for advancing our understanding of open-endedness. Complex systems science and the newest generation high-level programming languages provide intriguing possibilities to do so. Here, some recent advances in modeling and implementing open-ended evolutionary systems are reviewed (an earlier and shorter version was presented at [1]). Then, the so-called allagmatic method is introduced as a computational framework that describes, models, implements and allows interpreting complex systems using system metamodeling. Based on recent advances, the model building blocks evolving entities, entity lifetime parameter, co-evolutionary operations of entities and environment and combinatorial interactions are identified to characterize open-ended evolutionary systems. They are formalized within the system metamodel, providing a formal description of an open-ended evolutionary system. The study further provides a self-modifying code prototype in C# and guidance to create code blocks for an intrinsic implementation of open-ended evolutionary systems. This is achieved by controlling the self-modification of program code within the abstractly defined building blocks of the system metamodel. It is concluded that the identified model building blocks and the proposed self-modifying code provide a promising starting point to model and implement open-endedness in a computational system that potentially allows us to interpret novelties at runtime.
Keywords: Open-Ended Evolutionary Systems; Metamodeling; Self-Modifying Code; Combinatorial Evolution; Allagmatic Method
Cite this publication as:
P. Christen, “System Metamodeling of Open-Ended Evolution Implemented with Self-Modifying Code,” Complex Systems, 32(4), 2024 pp. 353–380.
https://doi.org/10.25088/ComplexSystems.32.4.353
An Alternative Representation of Turing Machines by Means of the Iota-Delta Function
Luan Carlos de Sena Monteiro Ozelim, André Luís Brasil Cavalcante, Todd Rowland and Jan M. Baetens
The evolution of universal systems has been of great interest to computer scientists. In particular, the role of Turing machines in the study of computational universality is widely recognized. Even though the patterns emerging from the evolution of this kind of dynamical system have been studied in much detail, the transition functions themselves have received less attention. In the present paper, the iota-delta function is used to encode the transition function of one-head Turing machines. In order to illustrate the methodology, we describe the transition functions of two universal Turing machines in terms of the latter function. By using the iota-delta function in this setting, Turing machines can be represented as a system of transition functions. This new representation allows us to write the transition functions as a linear combination of evolution variables wrapped by the iota-delta function. Thus, the nonlinear part of the evolution is totally described by the iota-delta function.
Keywords: Turing machines; universality; iota-delta function
Cite this publication as:
L. C. S. M. Ozelim, A. L. B. Cavalcante, T. Rowland and J. M. Baetens, “An Alternative Representation of Turing Machines by Means of the Iota-Delta Function,” Complex Systems, 32(4), 2024 pp. 381–393.
https://doi.org/10.25088/ComplexSystems.32.4.381
Formal Grammars Generating Fractal Descriptions of Molecular Structures
Savino Longo
Simple rewriting rules are used to produce alphanumeric strings that embed fractal number sequences and are directly translatable into descriptions of hydrocarbon structures of considerable complexity, featuring hierarchical schemes. Rotations of the alphanumeric strings lead to radical rearrangements of the corresponding structures, which lose their initial schemes and become much less predictable, featuring different topologies of polygonal cycles. This shows that a complex and not necessarily ordered molecular structure may nevertheless have a relatively low algorithmic complexity. The variety and versatility of reorganization in chemical topology, due to the nonlocal representation of bonds in the coding string, may have played a role in prebiotic chemistry.
Keywords: molecular modeling; formal languages; generative grammars; polycyclic alkanes; L-systems; algorithmic complexity; fractals
Cite this publication as:
S. Longo, “Formal Grammars Generating Fractal Descriptions of Molecular Structures,” Complex Systems, 32(4), 2024 pp. 395–407.
https://doi.org/10.25088/ComplexSystems.32.4.395
An Invitation to Higher Arity Science
Carlos Zapata-Carratalá and Xerxes D. Arsiwalla
Analytical thinking is dominated by binary ideas. From pairwise interactions to algebraic operations, to compositions of processes, to network models, binary structures are deeply ingrained in the fabric of most current scientific paradigms. In this paper, we introduce arity as the generic conceptualization of the order of an interaction between a discrete collection of entities and argue that there is a rich universe of higher arity ideas beyond binarity waiting to be explored. To illustrate this, we discuss several higher-order phenomena appearing in a wide range of research areas, paying special attention to instances of ternary interactions. From the point of view of formal sciences and mathematics, higher arity thinking opens up new paradigms of algebra, symbolic calculus and logic. In particular, we delve into the special case of ternary structures, as that itself reveals ample surprises: new notions of associativity (or lack thereof) in ternary operations of cubic matrices, ternary isomorphisms and ternary relations, the integration problem of 3-Lie algebras, and generalizations of adjacency in 3-uniform hypergraphs. All these are open problems that strongly suggest the need to develop new ternary mathematics. Finally, we comment on potential future research directions and remark on the transdisciplinary nature of higher arity science.
Keywords: higher-order structures; complex systems; interdisciplinary research; generalized associativity; ternary algebra; hypergraphs; matrix algebra; category theory
Cite this publication as:
C. Zapata-Carratalá and X. D. Arsiwalla, “An Invitation to Higher Arity Science,” Complex Systems, 32(4), 2024 pp. 409–468.
https://doi.org/10.25088/ComplexSystems.32.4.409
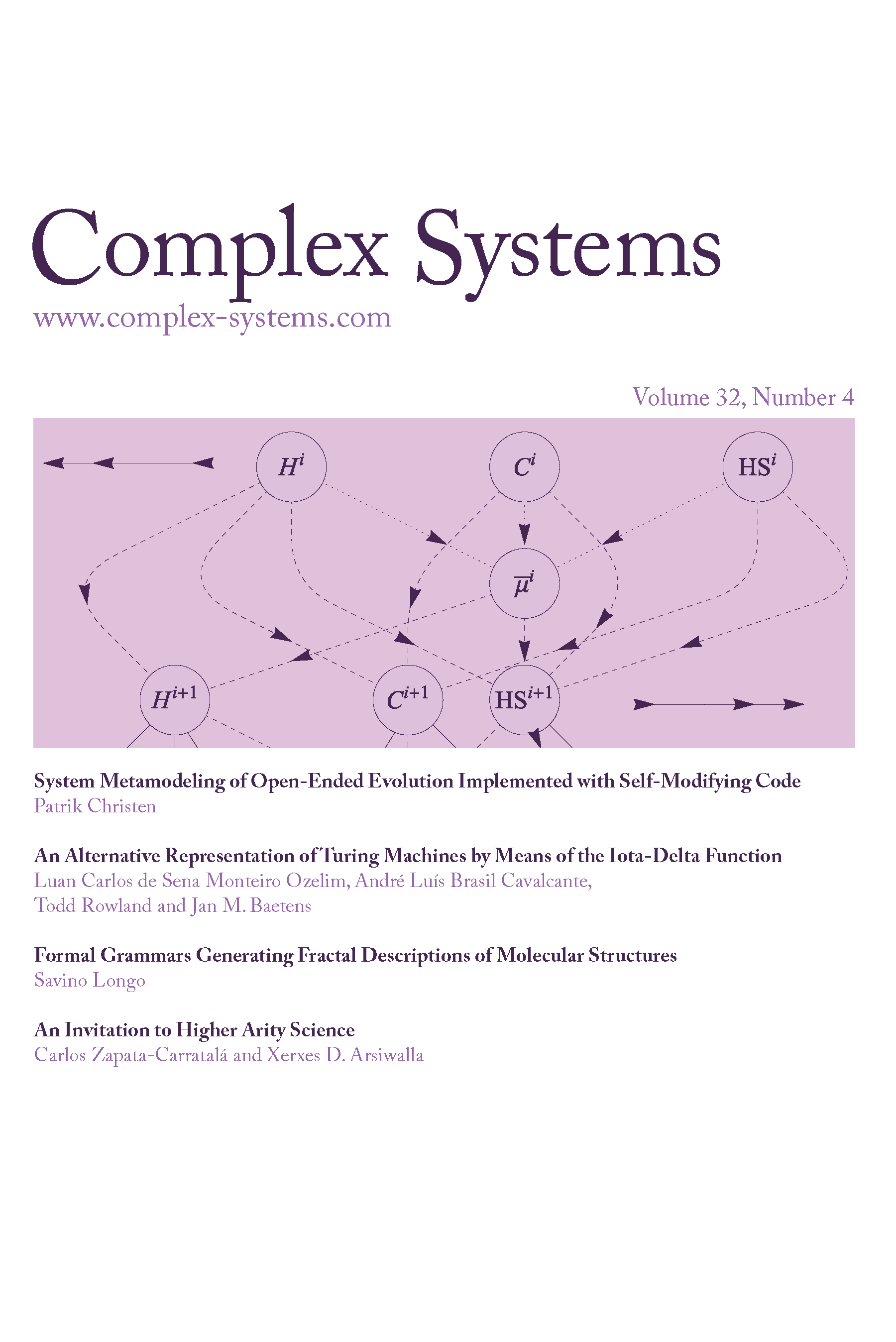

Complex Systems ISSN 0891-2513
© 1987–2024
Complex Systems Publication, Inc.
Published four times annually
Complex Systems Publications, Inc.
P.O. Box 6149
Champaign, IL 61826 USA

Join the leading edge of complex systems research today. There are no publication charges. Authors are provided with 25 reprints of papers published in Complex Systems. Papers may be submitted via the web or email. Find out more »